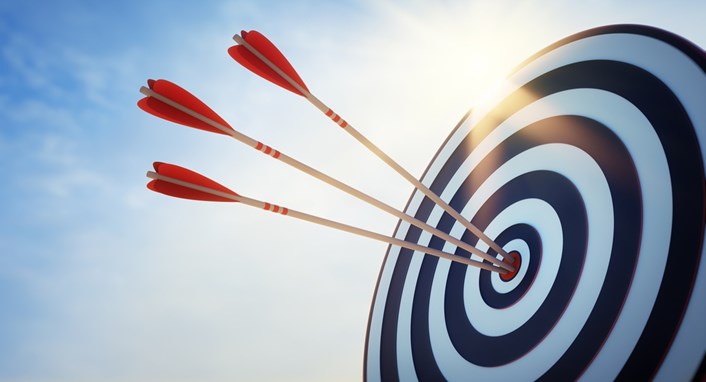
Maximizing debt collection with advanced scoring models
Debt collection plays a vital role in many industries, including banking, automotive, telco and utilities. Effective collection strategies are essential; without them the risk of default and financial loss increases significantly. This is especially true for lending institutions that need to recover outstanding debts from borrowers.
One the most effective ways to boost debt recovery is the use of advanced scoring models. These allow financial institutions to segment borrowers based on risk and the likelihood of repayment, enabling more targeted and efficient recovery strategies. Advanced models streamline debt collection and improve recovery rates, ensuring more sustainable financial operations.
The basics of debt collection
Debt collection involves a structured process aimed at recovering overdue payments from borrowers who have failed to meet their financial obligations. It plays a vital role in maintaining a healthy cash flow for financial institutions and minimizing potential losses.
Key components of this process include clear communication with borrowers, negotiating manageable repayment plans, and ensuring compliance with local debt collection regulations.
Clear documentation is also crucial, as it keeps a detailed record of interactions, agreements and any payment arrangements made with borrowers. By following these steps, lenders can not only protect their financial interests but also work toward maintaining positive relationships with their customers, ensuring long-term success.
Effective scoring models for debt collection
Advanced scoring models are key to improving debt collection efficiency. They provide a better assessment of borrowers based on specific factors such as their payment history, the number of accounts with outstanding payments, the average time they take to settle debts, and the total amount owed.
Some of the most effective models include:
- Risk segmentation. Borrowers are grouped according to their likelihood to repay. Higher-risk groups may require stronger and more immediate collection actions, while lower-risk borrowers can be managed using less aggressive strategies.
- Payment trend analysis. This involves examining historical payment behaviors to predict future actions, enabling lenders to adapt their recovery strategies accordingly.
- Average collection period assessment. By measuring how long it typically takes a borrower to repay a debt, financial institutions can estimate the potential risk and tailor their efforts.
- Outstanding balance evaluation. Analyzing the total amount owed helps lenders prioritize cases based on the financial impact of recovery.
By leveraging these scoring techniques, financial institutions can allocate resources more efficiently and maximize recovery rates, improving overall debt management.
How scoring improves debt collection efficiency
Advanced scoring models help segment borrowers and improve the operational efficiency of debt collection. By automating the evaluation of key factors such as payment history and outstanding balances, financial institutions can prioritize cases based on urgency and risk. This allows collection teams to focus their resources where they are needed most, reducing the time spent on low-priority accounts and enhancing overall productivity.
Furthermore, scoring helps take a more proactive approach to debt management. By predicting potential defaults, lenders can intervene early, offering tailored solutions before debts escalate.
This early action reduces the likelihood of write-offs and minimizes the costs associated with lengthy collection processes. Scoring also helps streamline communication strategies, ensuring that the appropriate tone and level of action are applied to each case, leading to better outcomes with less strain on resources.
Challenges and best practices in implementing scoring models
Despite the benefits of advanced scoring models, implementation can sometimes come up against obstacles. One key challenge is ensuring data accuracy. Without reliable, up-to-date information, scoring results may be flawed, leading to ineffective collection strategies.
Integration with existing systems can also pose technical difficulties, especially if platforms lack compatibility or require employees to navigate multiple systems.
Another potential barrier is the lack of automation within the collection management process. This can be overcome using a decision engine, which helps organizations to automate the collection decision by calculating a repayment plan with settlement option.
To address these challenges, regular data audits are essential to maintain accuracy and completeness. What’s more, choosing user-friendly solutions that integrate seamlessly with current systems can help avoid inefficiencies. Adequate staff training is crucial to ensure they understand the scoring results and can act accordingly. By following these best practices, financial institutions can optimize the effectiveness of scoring techniques and improve overall debt recovery.