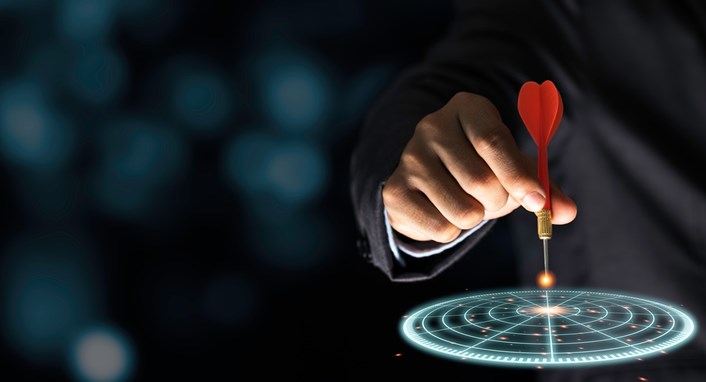
Enhancing credit profiling through effective categorization
In the complex landscape of financial decision making, credit profiling plays a pivotal role in determining the creditworthiness of individuals. This technique allows lending institutions to assess potential risks and make decisions that align with their business objectives.
As the financial sector continues to evolve, the accuracy of categorization in credit scoring has emerged as a crucial element that can significantly enhance the predictive power of credit risk models. Understanding how categorization influences credit profiling is essential for both financial institutions and consumers as they navigate their credit options.
Understanding data categorization
Data categorization involves the systematic classification of information into distinct groups, making it easier to analyze and interpret. In the context of credit scoring, categorization plays a fundamental role in transforming raw financial data into meaningful insights.
While it is possible to generate credit scores using only financial variables without categorization, this approach has its limitations. Purely financial interpretations may overlook subtleties in consumer behavior, such as the distinction between spending and saving.
By using categorization, financial institutions can better understand transaction patterns, enabling them to identify critical factors influencing an individual’s financial health.
For instance, categorization engines enable lenders to pinpoint essential income and expenditure categories – such as housing costs or existing debts – providing a clearer picture of a credit applicant's financial situation. This nuanced approach enhances the overall reliability of credit profiles, paving the way for more informed lending decisions.
The impact of accurate categorization on credit decisions
Accurate categorization is key to improved credit decisions, as it directly influences several key aspects of credit profiling. Firstly, it enhances the creditworthiness assessment by enabling lenders to better identify income and expenditure. This clarity provides critical indicators such as debt-to-income ratios and essential living expenses, which are indispensable for evaluating an individual’s ability to repay loans.
Secondly, accurate categorization helps to identify significant credit events or "red flags" that may indicate financial stress. By analyzing account transaction data, lenders can detect signs of potential credit default, enabling them to avoid lending to individuals already experiencing financial difficulties.
Lastly, accurate categorization feeds into the variables that contribute to credit scoring. This integration of advanced data enhances the predictive capabilities of credit scoring models, allowing lenders to more accurately estimate the likelihood of an applicant fulfilling their credit obligations. As a result, financial institutions can make evidence-based decisions, ultimately reducing their credit risk compared to traditional scoring methods.
Advanced credit scoring techniques
The advent of big data and advances in computing power have revolutionized credit scoring techniques, particularly through the integration of artificial intelligence (AI) and machine learning (ML).
These innovative methodologies significantly enhance the categorization process by enabling the analysis of vast amounts of unstructured data. With proprietary algorithms, financial institutions can transform raw transaction data into structured insights, providing a deeper understanding of consumer behavior.
Key techniques in advanced credit scoring include:
- Machine learning algorithms. These continuously learn from new data, adapting to changes in consumer spending patterns and economic conditions, thus improving the accuracy of categorization.
- Ensemble methods. By combining multiple classification techniques, ensemble approaches enhance overall performance, leveraging the strengths of various models for more robust outcomes.
- Predictive analytics. Advanced algorithms analyze historical data to identify trends and predict future behavior, enabling lenders to make data-driven decisions based on comprehensive risk assessments.
The future of credit profiling
The future of credit profiling will likely be driven by advances in data categorization and technology. Real-time data analysis will enhance the accuracy of credit assessments, allowing lenders to respond quickly to changes in a consumer’s financial situation.
What’s more, incorporating alternative data sources, such as utility bill and rent payments, could provide a more comprehensive view of creditworthiness, benefiting underbanked populations.
Regulatory developments will also shape credit profiling, as compliance with consumer privacy guidelines becomes paramount. Ultimately, prioritizing categorization accuracy will enable financial institutions to improve their decision-making processes and facilitate better outcomes for both lenders and consumers.