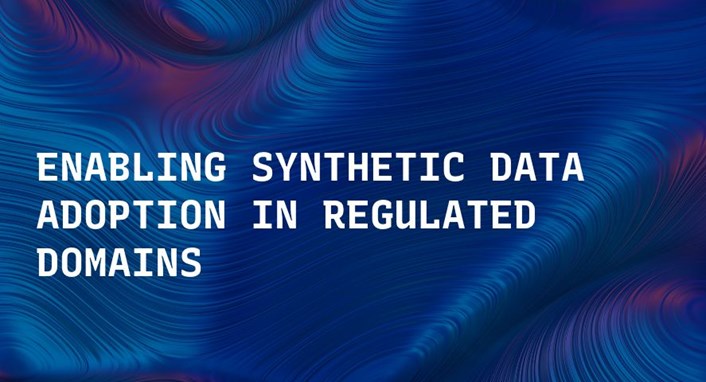
Enabling Synthetic Data adoption in regulated domains
Giorgio Visani, Giacomo Gra, Mattia Alfero, Enrico Bagli, Davide Capuzzo, and Federico Chesani
Abstract of Enabling Synthetic Data adoption in regulated domains
The switch from a Model-Centric to a Data-Centric mindset is putting emphasis on data and its quality rather than algorithms, bringing forward new challenges. In particular, the sensitive nature of the information in highly regulated scenarios needs to be accounted for. Specific approaches to address the privacy issue have been developed, as Privacy Enhancing Technologies. However, they frequently cause loss of information, putting forward a crucial trade-o among data quality and privacy. A clever way to bypass such a conundrum relies on Synthetic Data: data obtained from a generative process, learning the real data properties. Both Academia and Industry realized the importance of evaluating synthetic data quality: without all-round reliable metrics, the innovative data generation task has no proper objective function to maximize. Despite that, the topic remains under-explored. For this reason, we systematically catalog the important traits of synthetic data. quality and privacy, and devise a speci c methodology to test them. The result is DAISYnt (aDoption of Arti cial Intelligence SYnthesis): a comprehensive suite of advanced tests, which sets a de facto standard for synthetic data evaluation. As a practical use-case, a variety of generative algorithms have been trained on real-world Credit Bureau Data. The best model has been assessed, using DAISYnt on the di erent synthetic replicas. Further potential uses, among others, entail auditing and netuning of generative models or ensuring high quality of a given synthetic dataset. From a prescriptive viewpoint, eventually, DAISYnt may pave the way to synthetic data adoption in highly regulated domains, ranging from Finance to Healthcare, through Insurance and Education.
INTRODUCTION to Enabling Synthetic Data adoption in regulated domains
Critical aspects of a valuable dataset are data quality and privacy. The former is stressed in the Data-Centric mindset pioneered by Andrew Ng, while the latter is required by novel regulations such as the GDPR and the U.S. FERPA and HIPAA, educational and medical data privacy respectively. Privacy Enhancing Technologies already help protecting sensitive data, at the cost of an information loss. In fact, privacy and data quality behave as two antagonistic features. A clever way to potentially avoid such conflict relies on Synthetic Data: data obtained from a generative process, learning real data properties.
The quest for valuable synthetic data is highly relevant in regulated domains such as Finance and Healthcare, where they may enable several use-cases such as:
1) enforcing privacy protection,
2) facilitating data sharing among companies and towards the research community,
3) tackling class imbalance (eg. fraud detection),
4) increasing the amount of data for prediction models.
Despite that, the assessment of synthetic data quality and privacy remains an under-explored, although vital, topic. Whilst few taxonomies and tests have been proposed, we feel the need for a decisive improvement.
In this paper we tackle the open question of how to evaluate the quality and privacy of tabular synthetic data. Firstly, we systematically catalog their most important features into three concepts: Statistical Similarity, Data Utility and Privacy. To measure these notions, we devise appropriate state-of-the-art tests yielding a numeric value in the range , where higher metrics imply better performance. The final result is DAISYnt (aDoption of Artificial Intel-ligence SYnthesis): a comprehensive and easy to use test suite, that sets a de facto standard for synthetic data evaluation. As a practical use-case, a variety of generative algorithms have been trained on real-world Credit Bureau Data. The best model has been assessed, using DAISYnt on the different synthetic replicas. Further possible DAISYnt applications entail auditing and fine tuning of the models or ensuring high quality of a given synthetic dataset. In the following, Section 2 contains taxonomy and literature review. Section 3 is dedicated to general purpose tests, while Sections 4, 5 and 6 respectively concern with distribution similarity, data utility and privacy tests. Section 7 contains DAISYnt application on Credit Scoring data, while Section 8 contains a discussion on its implications and future perspectives. Methodological sections contain DAISYnt graphs and results on the Adult3 dataset from the UCI repos-itory.
ARE YOU A DEVELOPER?
Check out all the resources for TPPs and developers on the Crif Platform development portal.