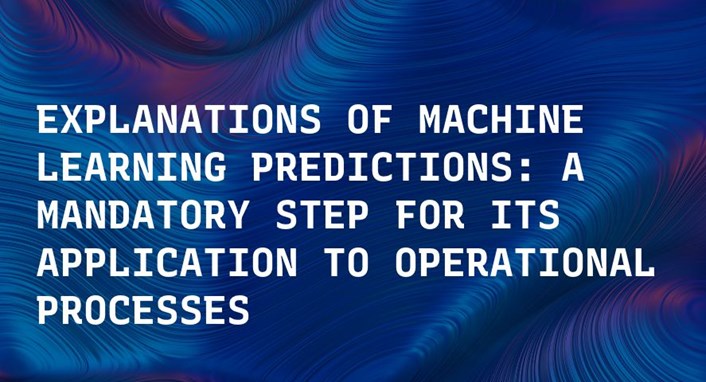
Explanations of Machine Learning predictions: a mandatory step for its application to Operational Processes
Giorgio Visani, Federico Chesani, Enrico Bagli, Davide Capuzzo and Alessandro Poluzzi
ABSTRACT of Explanations of Machine Learning predictions: a mandatory step for its application to Operational Processes
In the global economy, credit companies play a central role in economic development, through their activity of money lenders. This important task comes with some drawbacks, mainly the risk of the debtors of not being able to repay the provided credit. Therefore, Credit Risk Modelling (CRM), namely the evaluation of the probability that a debtor will not repay the due amount, plays a paramount role. Statistical approaches have been successfully exploited since long, becoming the most used methods for CRM. Recently, also machine and deep learning techniques have been applied to the CRM task, showing an important increase in prediction quality and performances. However, such techniques usually do not provide reliable explanations for the scores they come up with. As a consequence, many machine and deep learning techniques fail to comply with western countries regulations such as, for example, GDPR. In this paper we suggest to use LIME (Local Interpretable Model-agnostic Explanations) technique to tackle the explainability problem in this field, we show its employment on a real credit-risk dataset and eventually discuss its soundness and the necessary improvements to guarantee its adoption and compliance with the task.
Introduction to Explanations of Machine Learning predictions: a mandatory step for its application to Operational Processes
Operational Processes are defined as the core business of companies and firms: drug companies consider them to be drug testing and approval, manufacturing firms identify them in the product assembly process, while banks and financial firms have their own core business in risk management and evaluation. In order to be able to concede loans, financial institutions are compelled to predict whether an applicant is likely to repay the debit. In such a framework, Credit Scoring plays a huge role in ranking applicants based on their likelihood to pay back the loan. Each person is associated with a credit score value, namely a “number that summarizes its credit risk, based on a snapshot of its credit report at a particular point in time”. Behind the scenes, CRM is employed to reach the goal: scoring models, or “scorecards”, are generated from historical data, employing well-established statistical techniques. The cornerstones of a reliable scorecard are well depicted by Loretta Mester in: “the model should give a higher percentage of high scores to borrowers whose loans will perform well and a higher percentage of low scores to borrowers whose loans won’t perform well”. Several advantages stem from risk modelling, among the most important there are an increased profitability of financial corporations due to more reliable loans conceded, the chance of evaluating new loan programs based on the data collected and the enhancement of the credit-loss management capability. Therefore, over the years, some institutions arose to accomplish the task.
CRIF is a global company specialized in credit bureau and business information, outsourcing and processing services, and credit solutions. Its expertise in CRM dates back to the 80s, making the company one of the leaders in the Italian CRM market as well as an important benchmark worldwide. Nowadays, one of CRIF’s endeavours is towards the adoption of advanced analytics for CRM. This work represents a joint effort between CRIF and the University of Bologna. The aim is to exploit the state-of-the-art Machine Learning techniques and gain the benefits of their higher accuracy, while retaining the ability of producing reliable explanations about the models’ output. We consider it the first step to make such models adherent to the GDPR standards of UE and the more demanding countries. In this contribution, we introduce our approach, where classical (statistical) models are exploited alongside with Machine Learning ones, thus taking advantage of the enhanced accuracy. Then, we employ ground-breaking techniques, i.e. LIME, to achieve some interpretability of the outcomes. Prediction techniques and explanation capabilities are evaluated on a Credit Risk dataset. Eventually, we illustrate the approach by grounding it on few examples, showing the provided explanations, and discussing its reliability.
ARE YOU A DEVELOPER?
Check out all the resources for TPPs and developers on the Crif Platform development portal.