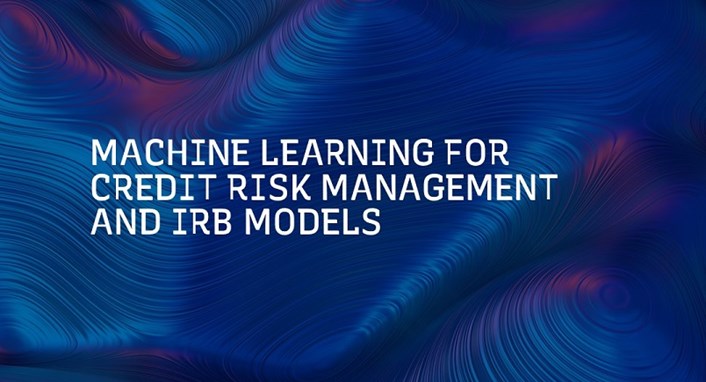
MACHINE LEARNING FOR CREDIT RISK MANAGEMENT AND IRB MODELS
Executive summary of MACHINE LEARNING FOR CREDIT RISK MANAGEMENT AND IRB MODELS
The use of machine learning (“ML”) models in banks has raised considerable interest and sparked a lively debate, among both scholars and practitioners. In November 2021, the European Banking Authority (“EBA”) has even published a discussion paper (“the Discussion Paper”) on the use of ML for internal ratings-based (“IRB”) models.
The Discussion Paper (European Banking Authority, 2021) notes that, in spite of their predictive power, ML models have not been incorporated into institutions’ IRB models as rapidly as in other areas. This is due to their complexity, and to the lack of a common understanding of their main characteristics and challenges when addressing regulatory requirements.
This has led the EBA to consider a set of principle-based recommendations to ensure that ML models comply with prudential provisions and do not harm the objective of implementing capital requirements in a prudent and consistent manner across Europe. Such recommendations include high-level requirements on data quality and model interpretability.
With reference to data quality, the use of unstructured data should not weaken the institutions’ ability to ensure accuracy, completeness and representativeness of the information used to feed ML models. As for interpretability, the management body and senior management should have a good understanding of ML models, including their key drivers and their impact on risk parameters; to achieve this goal, unnecessary complexity should be avoided, and an appropriate balance should be sought between a model’s performance and the explainability of its results.
The EBA has invited comments on the proposals put forward in the Discussion Paper. Having implemented ML models in banks for several years, we would like to use this opportunity to join the debate, providing the EBA and all interested parties with our views, based on past work in this field. We hope that this report – showing how the issues highlighted by the EBA were addressed in a number of real-life examples – will prove beneficial to the supervisors’ efforts to provide guidance on the ML-IRB relationship.
The remainder of this paper is structured as follows: in §2 we discuss the main opportunities and risks associated with ML models, providing a quick (and necessarily incomplete) classification, a picture of the main benefits they can provide to banks and a list of the possible pitfalls that need to be addressed; we then describe their current usage by financial institutions and review the main regulatory constraints to their development and usage as part of IRB rating systems. In §3 we present five case histories showing how ML has been used in banks, each time discussing the expected benefits and challenges, as well as data, algorithms, and interpretability techniques. In §4 we set out our conclusions.
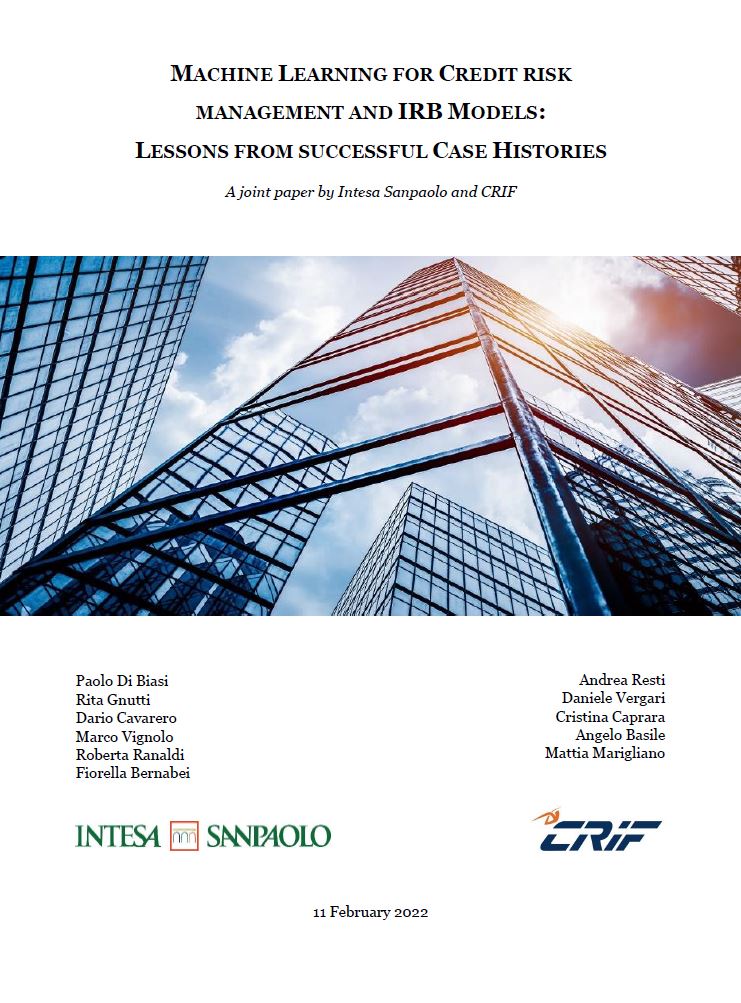